Carmine Rimi
on 12 August 2019
Digest #2019.08.12 – The Kubeflow Machine Learning Toolkit
- Kubeflow — a machine learning toolkit for Kubernetes – An introduction to Kubeflow from the perspective of a data scientist. This article quickly runs through some key components – Notebooks, Model Training, Fairing, Hyperparameter Tuning (Katib), Pipelines, Experiments, and Model Serving. If you are looking for a quick overview, give this article a go. Here’s a key diagram from the article:
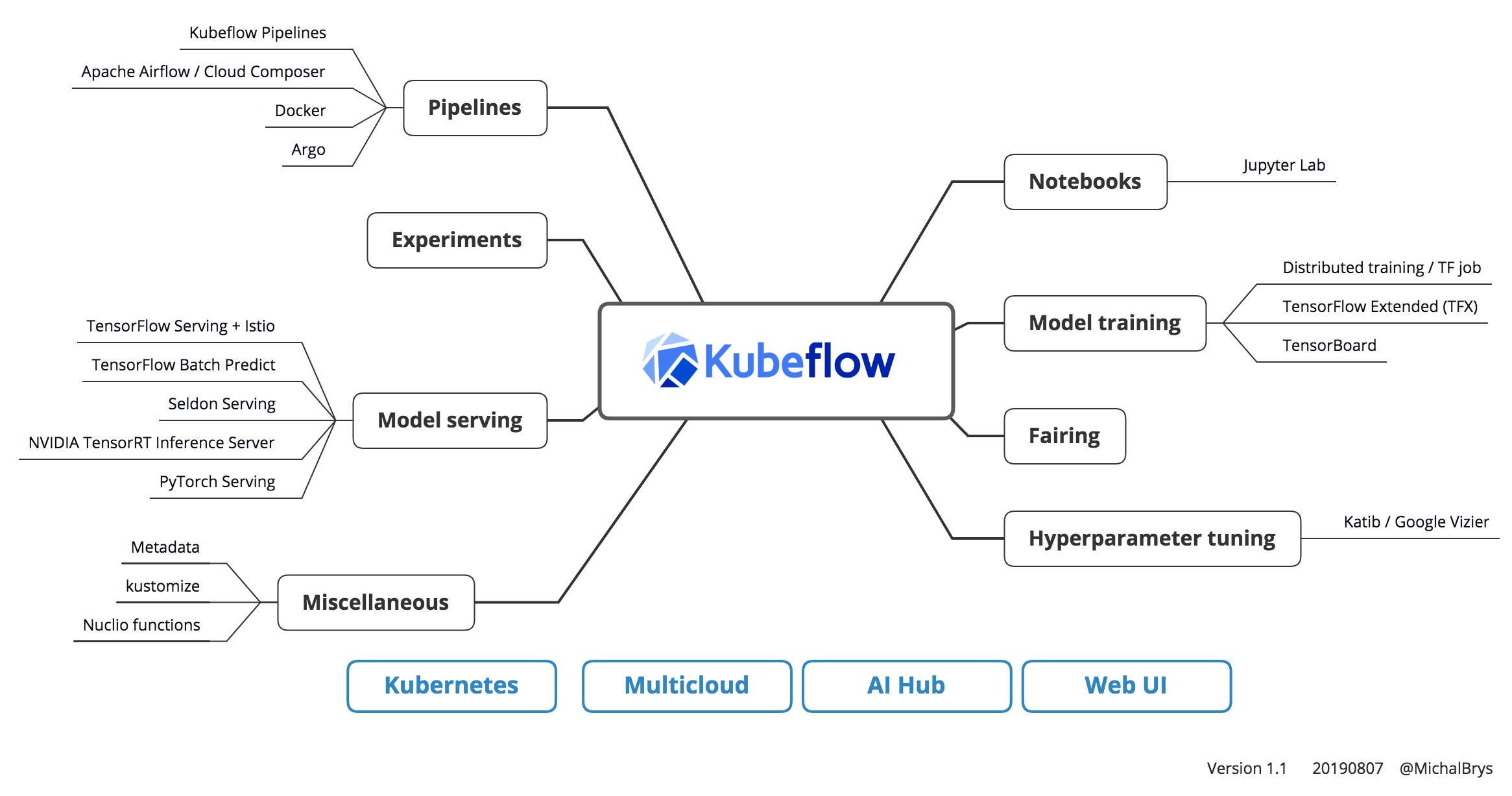
- Why is it So Hard to Integrate Machine Learning into Real Business Applications? – For teams just getting started, getting a trained model with sufficient accuracy is success. But that is just the starting point. There are many engineering and operational considerations that remain to be done. There are components that need to be built, tested and deployed. This post presents a real customer AI-based application, explaining some of the challenges, and suggests ways to simplify the development and deployment.
- Further afield – Techniques to improve the accuracy of your Predictive Models – a look at few techniques to improve the accuracy of your predictive models. The code base is in R, but the principles are applicable to a variety of code bases and algorithms.
- Use case spotlight – https://www.technologyreview.com/s/614043/instead-of-practicing-this-ai-mastered-chess-by-reading-about-it/. Instead of practicing, this AI mastered chess by reading about it. The chess algorithm, called SentiMATE, was developed by researchers at University College London. It evaluates the quality of chess moves by analysing the reaction of expert commentators. These learning techniques could have many other applications beyond chess – for instance, analysing sports, predicting financial activity, and making better recommendations.
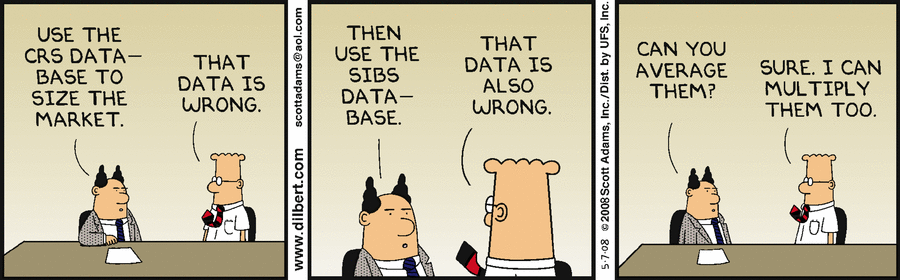